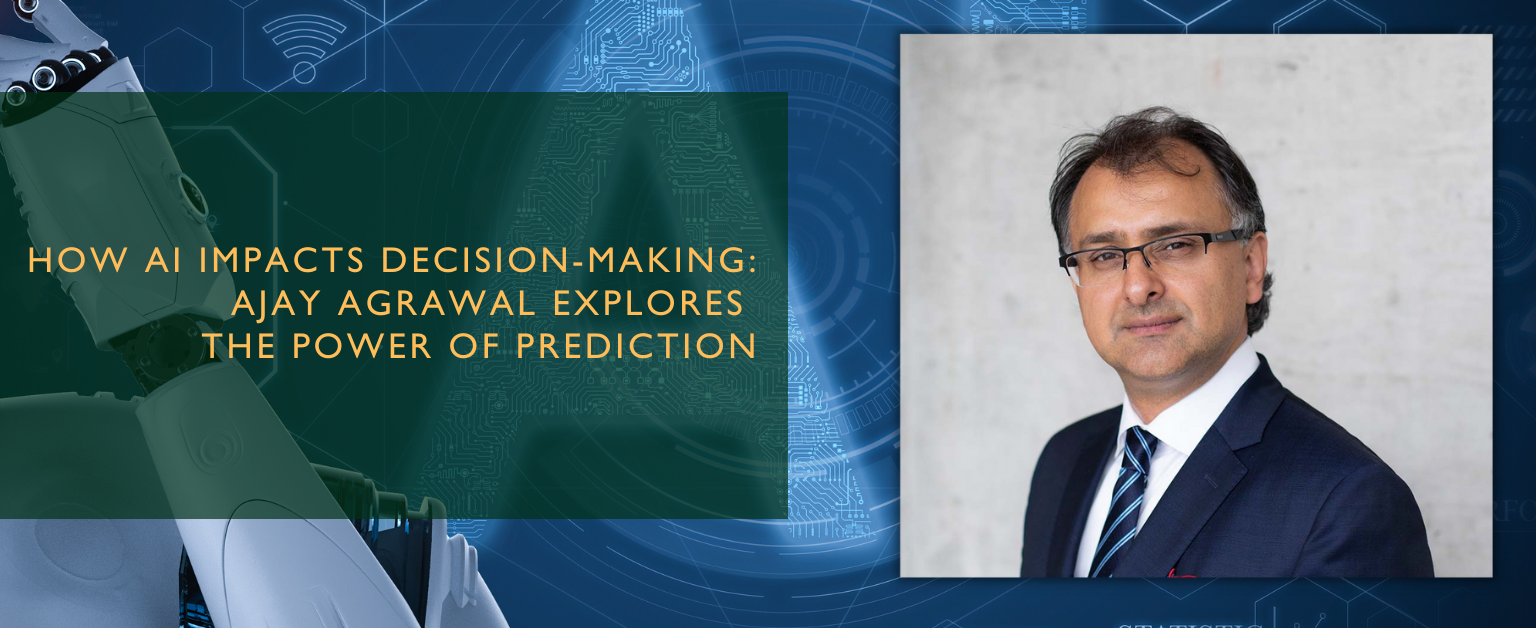
Thursday April 29th was not the first time Ajay Agrawal has been to Green College. The Richard V. Ericson lecturer from the Rotman School of Management at the University of Toronto was in fact visiting his old stomping grounds when he attended the Coach House to give a lecture on the economic disruptive capabilities of artificial intelligence (AI).
It was indeed fitting that Ajay revisited the College for the purpose of delivering the annual Richard V. Ericson lecture, as he was present to witness Richard Ericson’s important work in helping to found the College as its first Principal, not to mention being a remarkable scholar in his own right. As current Principal Mark Vessey remarked during his introduction, Ajay Agrawal was at the College right at the very beginning, in 1993, “when the first bunch of graduate students and others arrived to see about creating Green College with Principal Richard Ericson and the entire Ericson family.”
In opening his lecture, Ajay also referenced the indomitable Richard Ericson who presided over the foundations of Green College, accrediting the ongoing environment of collegiality and cross-disciplinary conversation to the decisions that he made in those early years. “One of the things that we are missing in academe is cross-disciplinary research and inquiry,” he remarked. “Universities are really unique environments where you get to pursue your curiosity. There are very few other occupations where you really get to do that, except that at universities it’s very disciplinary-oriented. I know it’s fashionable to talk about multidisciplinarity but very few places actually do it, but this was a place that did.”
Ajay’s talk centered on machine intelligence, a topic that draws on computer science, economics and statistics. In his lecture, he laid out three main themes, first establishing the foundational ideas in machine intelligence, before providing a structural explanation for the two waves of artificial intelligence (AI) adoption as well as arguing that we are only on the cusp of recognizing the power of AI and the responsibility we have to manage it.
The first foundational idea Ajay described was the supposition that the two key ingredients in decision-making are prediction and judgment. “Everything we do is riddled with decision-making,” he said, explaining that decision theory—a branch of probability theory—is concerned with how decisions are made by assigning probability to variables and numerical valuations to outcomes. As Ajay explained, decision-making is made up of two components: a probability assessment (how likely something is to occur or not occur) and a judgement (what we should do based on that likelihood). This process is something we do all the time, either consciously or unconsciously.
From an economist’s point of view, Ajay pointed out, the rise of AI can be seen as a more efficient route to probability assessments—as AI improves, the cost of making predictions falls. “So, we’ve got this technology that makes this thing cheaper, and the thing that is being [made] cheaper is foundational to decision-making,” he explained.
The upshot of outsourcing predication to the machine is a “decoupling of prediction from judgment.” By shifting the prediction aspect to machines, rather than human minds, we split the process of decision-making between AI and humans. “Just that decoupling—” he said, “you can think of it like splitting an atom—it is going to be the foundation of a whole bunch of transformations through the economy.”
Another foundational concept to understand about AI as a tool, is that it learns while you use it—the more you use it, the “smarter” it gets. AI doesn’t just make a prediction, it later discovers whether that prediction was accurate or not. If it was, the model is reinforced. And if it wasn’t, it updates the model. This means, essentially, that the more data accrued, the more accurate the predictions. This also means that AIs can very quickly become concentrated; a dominant AI becomes a more accurate AI, and in so doing reinforces its status as the primary tool of use. A perfect example is Google’s AI, which dominates the market for online searching, and by nature of this status, constantly receives the most data to update its model, thereby ensuring its domination of the field. The way that AI works is therefore oriented towards creating monopolies.
The third foundational concept Ajay presented was that when a general-use technology (that is, a technology that can be applied in many ways, think electricity or the internet) becomes cheaper, it has the capacity to have a significant social and economic impact by becoming accessible to whole new systems.
To explain this point, Ajay discussed the emergence of electricity as a general-use technology at the end of the nineteenth century. At first, the number of factories incorporating electricity was very small (a first wave, if you will). Sold primarily as a way of saving on energy costs, electricity was initially not a part of factories’ overall design, and it required modification to accommodate it. All that really changed in the first wave of implementation of electricity was a switch from one power source to another (that is, an impact at a single point in the factory system). As the technology became more common, however, a second wave of implementation of electricity can be traced: people began to construct factories that were more adaptable and more optimizable for machine operations—all of this because electricity allowed for the decoupling of machine from power source. Essentially, it became evident over time that electricity allowed not just for a replacement of the energy source, but a redesign of the entire system.
Taking these foundational ideas, Ajay presented the supposition that we are in the between times between the first and second waves of AI. What we have seen so far is the incorporation of AI at single points, but what is possible is the capacity for widespread systematic changes. “We think we are on the brink of a wave two,” he said.
The opportunities that may arise from this second wave, Ajay argued, can be seen by interpreting patterns using the foundational ideas outlined above. For example, the first AI “unicorn” in Canada, Ajay revealed, was not a start-up in Toronto, Montreal or Edmonton, where the big names in computer science are established. It was a start-up out of St. John’s called Verafin, an anti-financial crime firm that was acquired by Nasdaq for $2.75 billion in 2020. The reason that a financial AI firm was the first to reach this marker, he explained, was because financial industries are already investing resources in decoupling prediction from judgment, perhaps the most difficult jump in shifting to AI use.
A question presents itself, however: given all of these “smarter” systems, why has productivity so far remained stagnant? Building on the work of Erik Brynjolfsson, Daniel Rock and Chad Syverson (2017), Ajay suggests that what we are seeing is likely an implementation lag.
To explain this lagging pattern, Ajay returned to his analogy with electricity, recalling how the first wave was characterized by electricity simply being a way of doing one part of the process more cheaply—in this instance, producing energy. What we are doing with AI right now, he argues, is comparable: we are using it to make one part of the process—solving prediction problems where they have already been decoupled from judgement—more expediently and cost efficiently. What’s coming next, Ajay argued, is the system redesign, and this takes time and capital investment.
And this system change, while presenting profound opportunities for some, also presents significant risks to others, he noted. Touching on the potential for racial and gendered bias in AIs, Ajay also hinted at how the second wave could lead to a disruption to existing social power structures around the world.
Ajay Agrawal is the Geoffrey Taber Chair in Entrepreneurship and Innovation at the University of Toronto’s Rotman School of Management, Research Associate at the National Bureau of Economic Research in Cambridge, MA, Faculty Affiliate at the Vector Institute for Artificial Intelligence in Toronto, Board Member at Carnegie Mellon University’s Block Center for Technology and Society in Pittsburgh, PA, and Advisory Board Member for Green College. Ajay has published many scholarly articles on the economics of innovation and he is the co-author of Prediction Machines: The Simple Economics of Artificial Intelligence and co-editor of The Economics of Artificial Intelligence: An Agenda. Ajay serves on the editorial boards of Management Science and the Strategic Management Journal. He is the founder of the Creative Destruction Lab and co-founder of Next Canada, both of which are not-for-profit programs to support entrepreneurs. Ajay is also an alumnus of UBC and former Resident Member of Green College.
Ajay spoke at Green College on April 29, 2022 in a Richard V. Ericson Lecture titled "Power and Prediction: The Disruptive Economics of Artificial Intelligence." You can watch the recording here.
Post by: Jane Willsie, Department of English Language and Literature, UBC and Green College Work Learn Content Writer, 2022.